The application
Watcher is a simple productivity tool to check for changes on websites. Create individual watchers by setting the location (URL) for the page and path (XPath) for the content to monitor. The application then checks for any change in the content every 6 hours. It is an API first and API only application with 4 endpoints: create a watcher, list watchers, delete a watcher, test run a watcher.
Source code and deployment instructions for AWS are available on GitHub:designfultech/watcher-aws
Planning
I implemented the same application in Python running on Google App Engine, which gave a good baseline for comparison.
I was looking to build on a new architecture in a new environment. The application was going to be built on AWS in Node JS using as much of the AWS Serverless capabilities as possible.
AWS is in many ways the front-runner of Serverless and their Lambda service is one of the leading Function as a Service (FaaS) engine.
Node.js is a frequent choice of language for FaaS implementations and I wanted to see how it would compare to Python.
I was keen to use as many of the readily available functionality and services as possible, and write as little infrastructure code as possible (not to be confused with infrastructure as code).
Getting started
Serverless is full of infrastructure configurations, also known as infrastructure as code. It was a decision from the beginning that everything goes into code, no (Web) console monkey business in the final result. Wiring up and configuring the services in code (YAML) has a bit of a learning curve, and comes with a few surprises – more about these later. Production ready code requires a good amount of DevOps skills, there is no way around it with this architecture.
Other than picking the right language and suitable libraries there is not much else for the main logic. As for the infrastructure code, there are far too many options available.
- Native cloud scripting, templating
AWS has CloudFormation and its sister SAM - General purpose scripting and templating
Terraform and many others - Frameworks for manipulating the platform directly
Sceptre, Stacker, Troposphere - A range of toolkits specialising on Serverless and FaaS
Serverless Framework, Claudia.js and many others
Approach to infrastructure as code
The choice was to go with SAM and drop into CloudFormation where necessary.
Ultimately all the solutions will have a straight line down to the platform SDK.
The main question with 3rd party solutions is, how well and close they can follow platform changes? Do they support the entire platform and all the attributes needed?
Operating a platform in actual code lacks abstraction and too verbose.
Native platform scripts and templates offer the right level – close enough to the platform SDK for fine grain control, but well above in abstraction to be manageable. SAM as a bonus can raise the level of abstraction further to make life even easier. If there is a future for infrastructure as code it must be to do more with less code.
Other projects with different conditions may find the alternative solutions more suitable.
- A quick prototype probably makes better use of a toolkit like Serverless Framework.
- A large scale, multi-provider cloud environment could use a general purpose engine like Terraform.
- An adaptive environment with lots of logic in provisioning resources would need a coding approach like Troposphere.
Designing the application
Given the service oriented nature of Serverless and FaaS, a service definition seems to be a good start. Swagger (OpenAPI) is a good specification and has good platform support on AWS and in general. Another reason for choosing SAM templates was the reasonable level of support for Swagger.
Swagger specifics
- SAM does not handle multi-part Swagger files at the time of writing this article, which would be an issue for a larger project. One solution could be to use a pre-processor, something like Emrichen which looks very promising.
- Unfortunately the platform specifics and infrastructure as code leaks into the API definition, there is no easy and clean way around it. Therefore the Swagger definition is peppered with
x-amazon-
tags in support of the AWS platform and SAM. Perhaps the right pre-processor could merge external, platform-specific definitions in an additional step during deployment.
Platform services
Here is a high-level diagram of the AWS platform services in use in the application. Lot more relevant bits could go onto this diagram that are not trivial at first – more about these later.
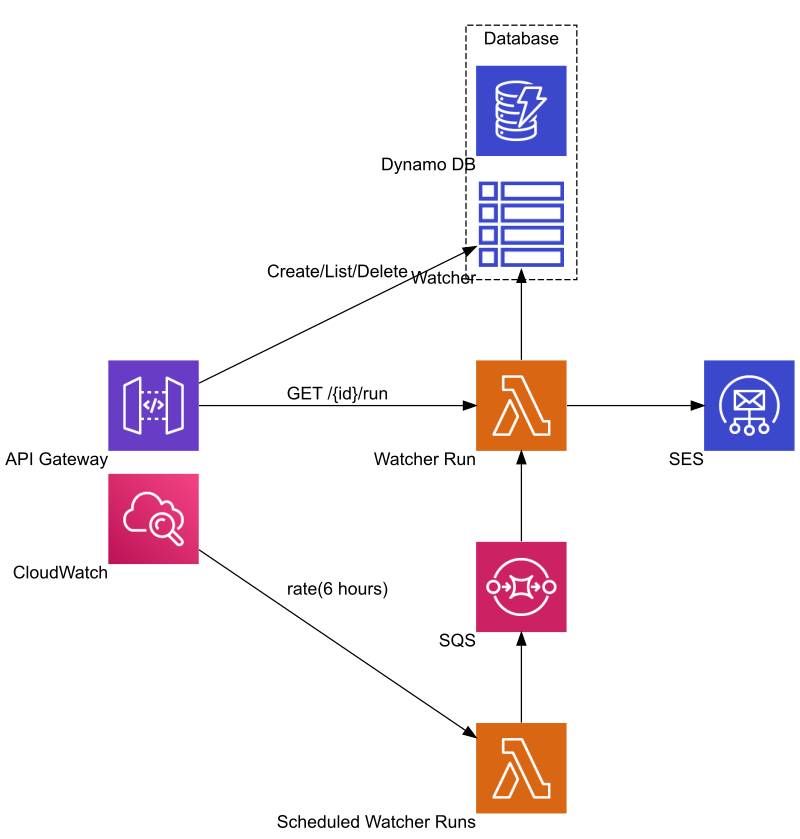
API Gateway was a given for reaching the REST API-s in the application.
DynamoDB can easily meet the database needs. It is also easy to expose CRUD + Query functions via the API Gateway.
Lambda was the primary candidate for the two services doing most of the work for the application.
CloudWatch has a scheduler function that comes in handy for triggering the regular checks.
Fanning out the individual checks can be done using a queue mechanism, SQS does the job well.
Finally, notification e-mails go out via SES.
Cross-cutting concerns
This project was specifically avoiding getting into cross-cutting concerns such as application configuration, security, logging, monitoring. They will be explored in another project.
Application configuration uses environment variables set at deployment time.
Security uses simple API key authentication. The implementation uses IAM’s roles and policies to authorise access, but it does not follow any recognisable model or method at this time.
Logging and monitoring was not explored in any detail. The first impressions of the vanilla logging facility were poor. The lack of out of box aggregated logging is a big surprise.
Building the application
Database (as a Service)
DynamoDB is a versatile key-value store with built-in REST endpoints for manipulating the data. I was keen to make use of the built-in capabilities and see how far they stretch. While DynamoDB does the job for this project, it will be increasingly more laborious to use for even slightly more complex jobs.
My very first task was to hook up DynamoDB operations to the API Gateway and be able to create new items (records), list them, and delete them without involving Lambda. It is all configurable in Swagger with relative ease.
Observations
- I could not return the newly created item in the response to the client. There is no support for
ALL_NEW
orUPDATED_NEW
inReturnValues
for theputItem
operation, so now the API returns an empty object with a 201 status. - Mapping templates (request and/or response) in Swagger look like code in the wrong place.
- Mapping actually uses another template language – Velocity. An immediate limitation I hit was trying to add a timestamp to the
created_at
attribute. There is no way to insert an ISO8601 UTC timestamp. The solution was to use$context.requestTime
, which is CLF-formatted. The only other alternative would be Epoch.
AWS Lambda in JavaScript (Node)
There are two functions implemented on Amazon Lambda:
- Checking a single item, web page, for any change.
- Periodically getting the list of watcher items and launching individual checks.
The code is fairly simple and self-explanatory for both functions, see comments in source code for more detail on GitHub.
Coding Lambda functions in Node/JavaScript was a breeze. Adopting the function*() yield combo in the programming model makes it very easy, sync-like, to code in this inherently async environment. Defining the functions in SAM is straight-forward.
I managed to focus on coding the application features without much distraction from infrastructure code. The one task that did not work well was manipulating data in DynamoDB. AWS SDK is too low level, it would not be efficient to use on a larger, more complex project. Next time I would definitely look for a decent ORM library, an abstraction on top of the SDK, something like Dynamoose.
The AWS SDK is bundled with the Lambda runtime. It does not need to be a dependency in the package.json, it can be a development dependency only.
Application integration
Every once in a while – set to 6 hours by default – one of the Lambda functions retrieves the list of watcher items to check for any change. There are a few different strategies to overcome the restrictions of Lambda function timeout, fanning out the check function seemed an easy and cost effective way to go. Each item from the list is passed to an SQS queue in a message, then picked by the target function.
A few considerations:
- Messages are processed in batches of 1 to 10 depending on the configuration. This use case needed to process only 1 message at a time.
- If the check fails for any reason, the message is put back into the queue and retried after a short while. Set a maximum number of deliveries on the message to avoid countless failed function calls and associated costs.
Another control mechanism, for other use cases, is to set the expiration on undelivered messages. - Worth defining a dead letter queue where failed messages are delivered after all tries are exhausted or timed out.
- Queues resources are not available in SAM, had to use CloudFormation AWS::SQS::Queue definitions.
The wide range of event sources supported by Lambda is very powerful. Be aware that event sources can have very different looking event data and determining the source from either context or event is not trivial.
One of the Functions – run-watcher – can be invoked via API Gateway and via SQS message. These two sources have entirely different message schemas. A small code piece is responsible for detecting the source, based on event specific attributes and parsing the message accordingly. In an ideal world, the AWS infrastructure would provide explicit information about the source of the different events.
SAM’s intricacies
The aforementioned scheduling of periodic checks was done using the AWS CloudWatch Timer service. This was easy enough to configure in SAM as an event on the Function.
SAM’s ability to create resources as necessary is a double-edge sword. While it significantly reduces the amount of infrastructure as code, it also introduces surprises to those who are not intimately familiar with all the platform specific resources of their application.
The scheduler resource is a good example to investigate. In SAM the scheduler event was defined in 4 lines as
Timer:
Type: Schedule
Properties:
Schedule: rate(6 hours)
The CloudFormation equivalent would be something like this
WatcherScanScheduler:
Type: AWS::Events::Rule
Properties:
Description: Schedule regular scans of watchers
Name: WatcherScanScheduler
ScheduleExpression: rate(6 hours)
State: ENABLED
Targets:
-
Arn: !GetAtt WatcherScanFunction.Arn
Id: WatcherScanFunction
The SAM version is ultimately expanded to the same CloudFormation definition by the tool. Notice that in SAM there is no place for some of the properties found in the explicit CloudFormation definition. This is why many of the resources generated by SAM behind the scenes will show up with seemingly random names. A good summary of generated resources by SAM is on https://awslabs.github.io.
This behaviour is not limited to SAM, many 3rd party tools that use an abstraction on top of CloudFormation have the same effect.
Resources depend on other resources. In most cases dependencies are satisfied with inserting a reference to the resource using their name or their ARN.
When creating the individual resources part of a stack, some of the references may not resolve because the pertinent resource do not exist yet at the time. The resolution to this problem is to explicitly state dependencies in the template. The usage plan (AWS::ApiGateway::UsagePlan) in the SAM template is a good example to analyse.
WatcherApiUsagePlan:
Type: AWS::ApiGateway::UsagePlan
DependsOn: WatcherApiStage
Properties:
ApiStages:
- ApiId: !Ref WatcherApi
Stage: !Ref EnvironmentParameter
Description: Private Usage Plan
Quota:
Limit: 500
Period: MONTH
Throttle:
BurstLimit: 2
RateLimit: 1
UsagePlanName: PrivateUse
Trying to deploy the stack without the explicitly stated dependency DependsOn: WatcherApiStage
will fail with not finding the Stage which is automagically generated by the SAM template together with the API (AWS::Serverless::Api) later.
This case is further complicated by SAM’s abstraction of API resources and hidingthe Stage resource definition. Where is the name WatcherApiStage
coming from? It is generated by SAM as described at this link.
The SAM CLI has a validation function to run on the SAM template, and the Swagger API Definition. The current validator has little value, failing to catch even simple schema violations. The true validation of the template happens when CloudFormation attempts to build the stack. In the future, infrastructure as code must have the same level of syntax and semantic check support as other programming languages.
Cross-over between infrastructure as code and application code
Occasionally the the application code must share information with the infrastructure as code. For example: the ARN of the queue where the application sends messages to. In this case, SAM has an environment variable for the Function that picks up the queue ARN using a utility function (GetAtt). The environment variable is read during execution to get the queue ARN for sending messages. This works well in most cases where infrastructure definitions are made in one place only and picked up during deployment, and the references do not change after deployment.
Setting the region for SES is a similar case, but warrants a short explanation. SES is only available in 3 specific regions and the application must use one of those for sending e-mails even it is deployed elsewhere. There are no SES resources (AWS::SES::…) defined in this project, yet the SES region is defined in SAM as an environment variable. It could have been a configuration in application code, but since SES is an infrastructure element, the region configuration is best placed in SAM.
Summary
The application works, it does what it is supposed to. There were some trade-offs made and there are areas for improvement. But most importantly the architecture and the platform has huge potential.
Building the application was easy enough. Was it as easy as on Google App Engine? That is for the next time.